One of the deep truths that every endurance athlete has to discover somewhere along their journey is that tomorrow matters as much as today. Maybe you can pick up the pace or add a few more miles to your workout; whether you should depends on whether doing so will compromise the next day鈥檚 training. The real gains come from stacking up weeks and months of work, but finding the edge of what鈥檚 sustainable is an art鈥攆or now, at least.
The latest attempt to turn athletic recovery into a science comes from researchers at the University of Auckland鈥檚 Sports Performance Research Institute New Zealand. A team led by Jeffrey Rothschild tracked 43 endurance athletes for up to 12 weeks, feeding reams of data into a series of machine learning models in an attempt to figure out what factors predict workout recovery. The results, which are , offer some surprising insights on what matters and, just as importantly, what doesn鈥檛.
The prerequisite for a study like this is athletes who are really into the idea of collecting data on themselves. The group ranged from amateurs to professionals and was mostly triathletes along with runners, cyclists, and a few rowers. They had to log all their workouts on TrainingPeaks, use a wearable device to measure sleep and heart-rate variability (HRV), answer a four-question survey every morning about their subjective well-being, and track everything they ate. That last one is a big barrier for most people, so the study focused on people who were already regularly tracking their food intake with an app such as MyFitnessPal.
Predicting Workout Recovery
The goal of collecting all this data was to predict how participants would answer a simple question every morning: , ranging from 鈥渧ery poorly recovered鈥 to 鈥渧ery well recovered.鈥 The researchers fed more than three-dozen variables into their machine learning algorithms: straightforward ones like training duration and number of calories consumed, as well more convoluted ones like training monotony, which is the average training load over the past week divided by the standard deviation of that week鈥檚 daily training loads.
Here’s what the analysis concluded are the most important variables for predicting perceived recovery score (PRS) in the morning:
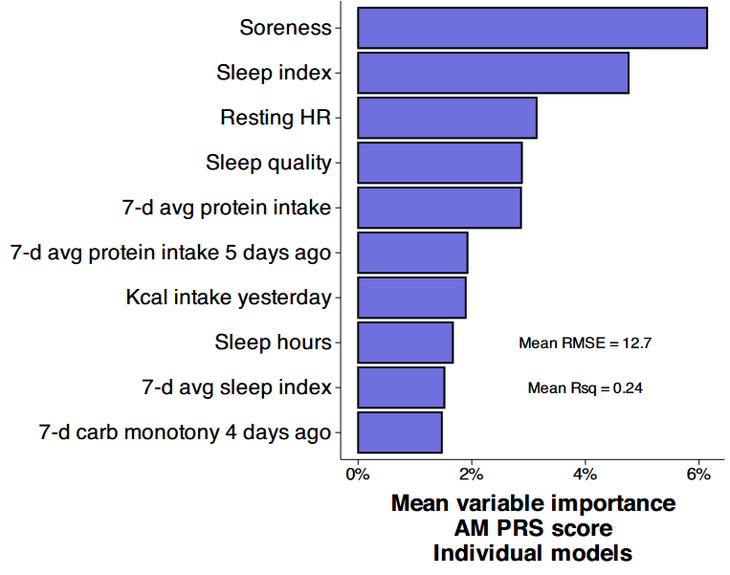
The biggest ones are soreness and sleep index, which was calculated by multiplying sleep duration by subjective sleep quality (i.e. if you sleep for seven hours and rate your sleep quality as 8 out of 10, your sleep index is 56). That makes intuitive sense: how you slept and how your first few steps out of bed feel dominate your perception of how ready you are to train again. After that, a bunch of other variables make modest contributions, like resting heart rate and how much protein you鈥檝e eaten in the past week.
The researchers also ran a simpler model using only soreness, sleep quality, perceived life stress score, and perceived recovery scores from the previous two days. This simpler model performed almost as well as the model with more than three-dozen inputs, suggesting that you don鈥檛 need to go nuts tracking everything.
There鈥檚 a big caveat, though. When they used their model on each individual athlete鈥檚 data, it didn鈥檛 do as well at predicting workout recovery. What worked better was to run a separate analysis of each athlete鈥檚 data to figure out which variables were most important to them. For example, one athlete鈥檚 recovery could be predicted very well using just sleep data from the prior night and the preceding week. Another athlete had their best predictions using muscle soreness, the previous day鈥檚 protein intake, and the previous day鈥檚 recovery score. It doesn鈥檛 take many variables to predict recovery, but those variables seem to be different for everyone.
What Predicts Heart-Rate Variability
Another key goal of the study was to determine which training and lifestyle variables predict changes in HRV, which is sometimes hyped as a simple one-stop-shop for assessing your recovery and readiness to train.
In this case, the picture was a little different. Here are the key variables ranked in order of importance to HRV changes:
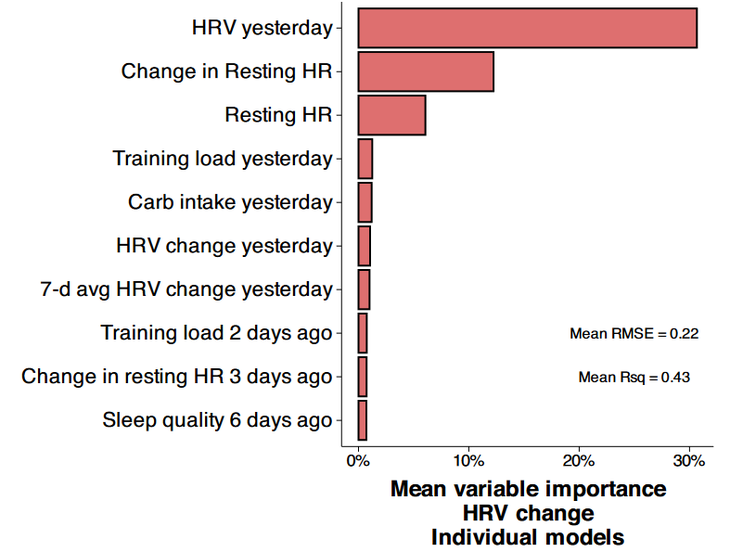
There鈥檚 one big variable that dominates the prediction of how much your HRV will change on a given day: how much it changed the previous day. If it increased yesterday, it鈥檚 likely to decrease today; if it decreased yesterday, it鈥檚 likely to increase today. The second and third variables both relate to resting heart rate, which also reflects how your automatic nervous system is doing. The rest of the variables鈥攕eemingly crucial data like how much you trained yesterday鈥攈ave much less impact.
Another part of the analysis involved trying to predict recovery and HRV using only 鈥渁ctionable鈥 variables鈥攖hat is, things that a coach or athlete could conceivably adjust in the course of a day of training and eating and sleeping. For example, you can adjust how long and hard you train, how much you eat, and how long you spend in bed. But you can鈥檛 adjust how well you sleep, or what your resting heart rate is.
The Limitations of HRV Prediction
Unfortunately, trying to predict HRV response using only actionable variables was basically futile. 鈥淔rom a practical perspective,鈥 Rothschild and his colleagues write, 鈥渦se of these models should be limited to communicating the expected values for an athlete on a given day, rather than suggesting ways to modulate the variables of interest.鈥 That reminds me (once again!) of the famous anecdote Nobel Prize-winning economist Kenneth Arrow told about his experiences as a weather forecaster during World War II. He realized that his long-range forecasts had no predictive value, and tried to warn his superiors. 鈥淭he Commanding General is well aware that the forecasts are no good,鈥 he was told. 鈥淗owever, he needs them for planning purposes.鈥
In other words, it鈥檚 not clear to me why we should measure HRV today if its main value is telling us what HRV will be tomorrow. Part of the problem, the authors note, is that the study ignored some variables that are known to influence HRV, like alcohol intake, illness, and menstrual cycle. Maybe a study with that data will produce better predictions.
For now, the biggest takeaway from the new data鈥攁nd it鈥檚 an important one鈥攊s that the factors that predict workout recovery are different for everyone. Sure, there are some broad patterns that apply to everyone, but some people may be totally wiped out by speed work, others by a bad night鈥檚 sleep, others by a too-small dinner. If you can tune into what those factors are for you鈥攅ither by running a machine-learning algorithm on a big pile of your own painstakingly collected data, or with old fashioned intuition and pattern-recognition鈥攜ou鈥檒l get the endurance athlete鈥檚 most coveted reward: to be able to train a little harder over the long term without burning out.
For more Sweat Science, join me on and , sign up for the , and check out my book .